Microbiome-wide association study
A microbiome-wide association study (MWAS), otherwise known as a metagenome-wide association study (MGWAS), is a statistical methodology used to examine the full metagenome of a defined microbiome in various organisms to determine if some feature (as example, gene or species) of the microbiome is associated with a host trait. MWAS has been adopted by the field of metagenomics from the widely used genome-wide association study (GWAS).
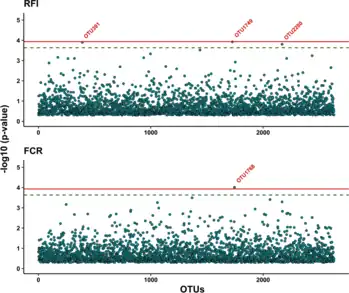
While MWAS is phonetically and conceptually tied to GWAS there are several key differentiations:
- There are roughly 150 times more genes in the microbiome than in the human genome.[2] A GWAS must only find significantly associated genes along the predefined number of chromosomes of the species. On the other hand, the MWAS must analyze however many features are in an undetermined number of microorganisms. As a result, there is a far higher chance of running into the multiple testing problem.[3]
- While host populations contain a relatively similar collection of genes on the genome, the genetic variation of any given microbiome can vary significantly between different hosts and environments.[4] The genome of the microbiome can also vary temporally in a given host [5] while the genome of the host in a GWAS is fixed across their lifespan.
- The realized microbiome datasets are inherently compositional [6] and interactional. The assumption that the genes exist in a Euclidean space is violated by the non-linear nature of compositional data.[7]
There are several ways to classify which feature of the microbiome will be used in a MWAS. MWAS can be assessed using a specific taxonomic level (species, genus,[8] phyla, etc.), operational taxonomic unit (OTU) [1] or amplicon sequence variant (ASV), transcriptome,[9] proteome,[10] and more. The approach used depends upon the research hypothesis as each method will often give differing results.
Often, a taxonomic level or OTU/ASV based approach is used to determine the correlations between the specific microbiome feature and the desired phenotype. Several methods can be employed, such as machine learning approaches like random forests,[11] and deep learning.[12] Feature association can also be established with programs like DESeq2 and ANCOM. However, correlations established by the wide array of tools available may not always translate into causality. Researchers determine causality through sequential testing.[13]
References
- Aliakbari, Amir; Zemb, Olivier; Cauquil, Laurent; Barilly, Céline; Billon, Yvon; Gilbert, Hélène (2022-04-25). "Microbiability and microbiome-wide association analyses of feed efficiency and performance traits in pigs". Genetics Selection Evolution. 54 (1): 29. doi:10.1186/s12711-022-00717-7. ISSN 1297-9686. PMC 9036775. PMID 35468740.
- MetaHIT Consortium; Qin, Junjie; Li, Ruiqiang; Raes, Jeroen; Arumugam, Manimozhiyan; Burgdorf, Kristoffer Solvsten; Manichanh, Chaysavanh; Nielsen, Trine; Pons, Nicolas; Levenez, Florence; Yamada, Takuji; Mende, Daniel R.; Li, Junhua; Xu, Junming; Li, Shaochuan (2010-03-04). "A human gut microbial gene catalogue established by metagenomic sequencing". Nature. 464 (7285): 59–65. Bibcode:2010Natur.464...59.. doi:10.1038/nature08821. ISSN 0028-0836. PMC 3779803. PMID 20203603.
- Gilbert, Jack A.; Quinn, Robert A.; Debelius, Justine; Xu, Zhenjiang Z.; Morton, James; Garg, Neha; Jansson, Janet K.; Dorrestein, Pieter C.; Knight, Rob (2016-07-07). "Microbiome-wide association studies link dynamic microbial consortia to disease". Nature. 535 (7610): 94–103. Bibcode:2016Natur.535...94G. doi:10.1038/nature18850. ISSN 0028-0836. PMID 27383984. S2CID 4455429.
- Koenig, Jeremy E.; Spor, Aymé; Scalfone, Nicholas; Fricker, Ashwana D.; Stombaugh, Jesse; Knight, Rob; Angenent, Largus T.; Ley, Ruth E. (2011-03-15). "Succession of microbial consortia in the developing infant gut microbiome". Proceedings of the National Academy of Sciences. 108 (supplement_1): 4578–4585. Bibcode:2011PNAS..108.4578K. doi:10.1073/pnas.1000081107. ISSN 0027-8424. PMC 3063592. PMID 20668239.
- Vandeputte, Doris; De Commer, Lindsey; Tito, Raul Y.; Kathagen, Gunter; Sabino, João; Vermeire, Séverine; Faust, Karoline; Raes, Jeroen (2021-11-18). "Temporal variability in quantitative human gut microbiome profiles and implications for clinical research". Nature Communications. 12 (1): 6740. Bibcode:2021NatCo..12.6740V. doi:10.1038/s41467-021-27098-7. ISSN 2041-1723. PMC 8602282. PMID 34795283.
- Gloor, Gregory B.; Macklaim, Jean M.; Pawlowsky-Glahn, Vera; Egozcue, Juan J. (2017-11-15). "Microbiome Datasets Are Compositional: And This Is Not Optional". Frontiers in Microbiology. 8: 2224. doi:10.3389/fmicb.2017.02224. ISSN 1664-302X. PMC 5695134. PMID 29187837.
- Aitchison, John; Greenacre, Michael (2002-10-08). "Biplots of compositional data". Journal of the Royal Statistical Society Series C: Applied Statistics. 51 (4): 375–392. doi:10.1111/1467-9876.00275. hdl:10230/1046. ISSN 0035-9254.
- Davenport, Emily R.; Cusanovich, Darren A.; Michelini, Katelyn; Barreiro, Luis B.; Ober, Carole; Gilad, Yoav (2015-11-03). White, Bryan A (ed.). "Genome-Wide Association Studies of the Human Gut Microbiota". PLOS ONE. 10 (11): e0140301. Bibcode:2015PLoSO..1040301D. doi:10.1371/journal.pone.0140301. ISSN 1932-6203. PMC 4631601. PMID 26528553.
- Huang, Hechen; Ren, Zhigang; Gao, Xingxing; Hu, Xiaoyi; Zhou, Yuan; Jiang, Jianwen; Lu, Haifeng; Yin, Shengyong; Ji, Junfang; Zhou, Lin; Zheng, Shusen (2020-11-23). "Integrated analysis of microbiome and host transcriptome reveals correlations between gut microbiota and clinical outcomes in HBV-related hepatocellular carcinoma". Genome Medicine. 12 (1): 102. doi:10.1186/s13073-020-00796-5. ISSN 1756-994X. PMC 7682083. PMID 33225985.
- Riddell, Nina; Crewther, Sheila G. (2017-01-30). "Integrated Comparison of GWAS, Transcriptome, and Proteomics Studies Highlights Similarities in the Biological Basis of Animal and Human Myopia". Investigative Ophthalmology & Visual Science. 58 (1): 660–669. doi:10.1167/iovs.16-20618. ISSN 1552-5783. PMID 28135361.
- Zajac, Diana J.; Green, Stefan J.; Johnson, Lance A.; Estus, Steven (2021-08-03). "APOE Genetics Influence Murine Gut Microbiome". doi:10.21203/rs.3.rs-746611/v1.
{{cite journal}}
: Cite journal requires|journal=
(help) - Zhu, Qiang; Li, Bojing; He, Tingting; Li, Guangrong; Jiang, Xingpeng (2020-02-15). "Robust biomarker discovery for microbiome-wide association studies". Methods. 173: 44–51. doi:10.1016/j.ymeth.2019.06.012. PMID 31238097. S2CID 195660885.
- Surana, Neeraj K.; Kasper, Dennis L. (2017-12-14). "Moving beyond microbiome-wide associations to causal microbe identification". Nature. 552 (7684): 244–247. Bibcode:2017Natur.552..244S. doi:10.1038/nature25019. ISSN 0028-0836. PMC 5730484. PMID 29211710.