Single-cell variability
In cell biology, single-cell variability occurs when individual cells in an otherwise similar population differ in shape, size, position in the cell cycle, or molecular-level characteristics. Such differences can be detected using modern single-cell analysis techniques.[1] Investigation of variability within a population of cells contributes to understanding of developmental and pathological processes,
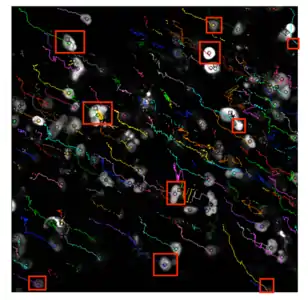
Single-cell analysis
A sample of cells may appear similar, but the cells can vary in their individual characteristics, such as shape and size, mRNA expression levels, genome, or individual counts of metabolites. In the past, the only methods available for investigating such properties required a population of cells and provided an estimate of the characteristic of interest, averaged over the population, which could obscure important differences among the cells. Single-cell analysis allows scientists to study the properties of a single cell of interest with high accuracy, revealing individual differences among populations and offering new insights in molecular biology. These individual differences are important in fields such as developmental biology, where individual cells can take on different "fates" - become specialized cells such as neurons or organ tissue - during the growth of an embryo; in cancer research, where individual malignant cells can vary in their response to therapy; or in infectious disease, where only a subset of cells in a population become infected by a pathogen.
Population-level views of cells can offer a distorted view of the data by averaging out the properties of distinct subsets of cells.[3] For example, if half the cells of a particular group are expressing high levels of a given gene, and the rest are expressing low levels, results from a population-wide analysis may appear as if all cells are expressing a medium level of the given gene. Thus, single-cell analysis allows researchers to study biological processes in finer detail and answer questions that could not have been addressed otherwise.
Types of variation
Variation in gene expression
Cells with identical genomes may vary in the expression of their genes due to differences in their specialized function in the body, their timepoint in the cell cycle, their environment, and also noise and stochastic factors. Thus, accurate measurement of gene expression in individual cells allows researchers to better understand these critical aspects of cellular biology. For example, early study of gene expression in individual cells in fruit fly embryos allowed scientists to discover regularized patterns or gradients of specific gene transcription during different stages of growth, allowing for a more detailed understanding of development at the level of location and time. Another phenomenon in gene expression which could only be identified at the single cell level is oscillatory gene expression, in which a gene is expressed on and off periodically.
Single-cell gene expression is typically assayed using RNA-seq. After the cell has been isolated, the RNA-seq protocol typically consists of three steps:[4] the RNA is reverse transcribed into cDNA, the cDNA is amplified to make more material available for the sequencer, and the cDNA is sequenced.
Variation in DNA sequence
A population of single celled organisms like bacteria typically vary slightly in their DNA sequence due to mutations acquired during reproduction. Within a single human, individual cells typically have identical genomes, though there are interesting exceptions, such as B-cells, which have variation in their DNA enabling them to generate different antibodies to bind to the variety of pathogens that can attack the body. Measuring the differences and the rate of change in DNA content at the single-cell level can help scientists better understand how pathogens develop antibiotic resistance, why the immune system often cannot produce antibodies for rapidly mutating viruses like HIV, and other important phenomena.
Many technologies exist for sequencing genomes, but they are designed to use DNA from a population of cells rather than a single cell. The primary challenge for single-cell genome sequencing is to make multiple copies of (amplify) the DNA so that there is enough material available for the sequencer, a process called whole genome amplification (WGA). Typical methods for WGA consist of:[5] (1) Multiple Displacement Amplification (MDA) in which multiple primers anneal to the DNA, polymerases copy the DNA, and knock off other polymerases, freeing strands that can be processed by the sequencer, (2) PCR-based methods, or (3) some combination of both.
Variation in metabolomic properties
Cells vary in the metabolites they contain, which are the intermediary compounds and end products of complex biochemical reactions that sustain the cell. Genetically identical cells in different conditions and environments can use different metabolic pathways to sustain themselves. By measuring the metabolites present, scientists can infer the metabolic pathways used, and infer useful information about the state of the cell. An example of this is found in the immune system, where CD4+ cells can differentiate into Th17 or TReg cells (among other possibilities), both of which direct the immune system's response in different ways. Th17 cells stimulate a strong inflammatory response, whereas TReg cells stimulate the opposite effect. The former tend to rely much more on glycolysis,[6] due to their increased energy demands.
In order to profile the metabolic content of a cell, researchers must identify the cell of interest in the larger population, isolate it for analysis, quickly inhibit enzymes and halt the metabolic processes in the cell, and then use techniques such as NMR, mass-spec, microfluidics, and other methods to analyze the contents of the cell.[7]
Variation in proteome
Similar to variation in the metabolome, the proteins present in a cell and their abundances can vary from cell to cell in an otherwise similar population. While transcription and translation determine the amount and variety of proteins produced, these processes are imprecise, and cells have a number of mechanisms which can change or degrade proteins, allowing for variance in the proteome that may not be accounted for by variance in gene expression. Also, proteins have many other important features besides simply being present or absent, such as whether have undergone posttranslational modifications such as phosphorylation, or are bound to molecules of interest. The variation in abundance and characteristics of proteins has implications for fields such as cancer research and cancer therapy, where a drug targeting a particular protein may vary in its impact due to variability in the proteome,[8] or vary in efficacy due to the broader biological phenomenon of tumor heterogeneity.
Cytometry, surface methods, and microfluidics technologies are the three classes of tools commonly used to profile the proteomes of individual cells.[9] Cytometry allows researchers to isolate cells of interest, and stain 15–30 proteins to measure their location and/or relative abundance.[9] Image cycling techniques have been developed to measure multi-target abundance and distribution in biopsy samples and tissues. In these methods, 3–4 targets are stained with fluorescently labeled antibodies, imaged, and then stripped of their fluorophores by a variety of means, including oxidation-based chemistries[10] or more recently antibody-DNA conjugation methods,[11] allowing additional targets to be stained in follow-on cycles; in some methods up to 60 individual targets have been visualized.[12] For surface methods, researchers place a single cell on a surface coated with antibodies, which then bind to proteins secreted by the cell and allow them to be measured.[9] Microfluidics methods for proteome analysis immobilize single cells on a microchip and use staining to measure the proteins of interest, or antibodies to bind to the proteins of interest.
Variation in cell size and morphology
Cells in an otherwise similar population can vary in their size and morphology due to differences in function, changes in metabolism, or simply being in different phases of the cell cycle or some other factor. For example, stem cells can divide asymmetrically,[13] which means the two resultant daughter cells may have different fates (specialized functions), and can differ from each other in size or shape. Researchers who study development may be interested in tracking the physical characteristics of the individual progeny in a growing population in order to understand how stem cells differentiate into a complex tissue or organism over time.
Microscopy can be used to analyze cell size and morphology by obtaining high-quality images over time. These pictures will typically contain a population of cells, but algorithms can be applied to identify and track individual cells across multiple images. The algorithms must be able to process gigabytes of data to remove noise and summarize the relevant characteristics for the given research question.[14]
Variation in cell cycle
Individual cells in a population will often be at different points in the cell cycle. Scientists who wish to understand characteristics of the cell at a particular point in the cycle would have difficulty using population-level estimates, since they would average measurements from cells at different stages. Also, understanding the cell cycle in individual diseased cells, like those in a tumor, is also important, since they will often have a very different cycle than healthy cells. Single-cell analysis of characteristics of the cell cycle allow scientists to understand these properties in greater detail.
Variability in cell cycle can be studied using several of the methods previously described. For example, cells in G2 will be quite large in size (as they are a just at the point where they are about to divide in two), and can be identified using protocols for cell size and shape. Cells in S phase copy their genomes, and could be identified using protocols for staining DNA and measuring its content by flow cytometry or quantitative fluorescence microscopy, or by using probes for genes expressed highly at specific phases of the cell cycle.
References
- Habibi, Iman; Cheong, Raymond; Lipniacki, Tomasz; Levchenko, Andre; Emamian, Effat S.; Abdi, Ali (2017-04-05). "Computation and measurement of cell decision making errors using single cell data". PLOS Computational Biology. 13 (4): e1005436. Bibcode:2017PLSCB..13E5436H. doi:10.1371/journal.pcbi.1005436. ISSN 1553-7358. PMC 5397092. PMID 28379950.
- Giedt, Randy J.; Koch, Peter D.; Weissleder, Ralph; Muñoz-Barrutia, Arrate (10 April 2013). "Single Cell Analysis of Drug Distribution by Intravital Imaging". PLOS ONE. 8 (4): e60988. Bibcode:2013PLoSO...860988G. doi:10.1371/journal.pone.0060988. PMC 3622689. PMID 23593370.
- Sandberg, Rickard (30 December 2013). "Entering the era of single-cell transcriptomics in biology and medicine". Nature Methods. 11 (1): 22–24. doi:10.1038/nmeth.2764. PMID 24524133. S2CID 27632439.
- Saliba, A.-E.; Westermann, A. J.; Gorski, S. A.; Vogel, J. (22 July 2014). "Single-cell RNA-seq: advances and future challenges". Nucleic Acids Research. 42 (14): 8845–8860. doi:10.1093/nar/gku555. PMC 4132710. PMID 25053837.
- Macaulay, Iain C.; Voet, Thierry; Maizels, Nancy (30 January 2014). "Single Cell Genomics: Advances and Future Perspectives". PLOS Genetics. 10 (1): e1004126. doi:10.1371/journal.pgen.1004126. PMC 3907301. PMID 24497842.
- Barbi, Joseph; Pardoll, Drew; Pan, Fan (March 2013). "Metabolic control of the Treg/Th17 axis". Immunological Reviews. 252 (1): 52–77. doi:10.1111/imr.12029. PMC 3576873. PMID 23405895.
- Rubakhin, Stanislav S; Romanova, Elena V; Nemes, Peter; Sweedler, Jonathan V (30 March 2011). "Profiling metabolites and peptides in single cells". Nature Methods. 8 (4s): S20–S29. doi:10.1038/nmeth.1549. PMC 3312877. PMID 21451513.
- Cohen, A. A.; Geva-Zatorsky, N.; Eden, E.; Frenkel-Morgenstern, M.; Issaeva, I.; Sigal, A.; Milo, R.; Cohen-Saidon, C.; Liron, Y.; Kam, Z.; Cohen, L.; Danon, T.; Perzov, N.; Alon, U. (5 December 2008). "Dynamic Proteomics of Individual Cancer Cells in Response to a Drug". Science. 322 (5907): 1511–1516. Bibcode:2008Sci...322.1511C. doi:10.1126/science.1160165. PMID 19023046. S2CID 9553016.
- Wei, Wei; Shin, Young; Ma, Chao; Wang, Jun; Elitas, Meltem; Fan, Rong; Heath, James R (2013). "Microchip platforms for multiplex single-cell functional proteomics with applications to immunology and cancer research". Genome Medicine. 5 (8): 75. doi:10.1186/gm479. PMC 3978720. PMID 23998271.
- Sorger, Peter K.; Fallahi-Sichani, Mohammad; Lin, Jia-Ren (2015-09-24). "Highly multiplexed imaging of single cells using a high-throughput cyclic immunofluorescence method". Nature Communications. 6: 8390. Bibcode:2015NatCo...6.8390L. doi:10.1038/ncomms9390. ISSN 2041-1723. PMC 4587398. PMID 26399630.
- Weissleder, Ralph; Juric, Dejan; Castillo, Andres Fernandez del; McFarland, Philip J.; Carlson, Jonathan C. T.; Pathania, Divya; Giedt, Randy J. (2018-10-31). "Single-cell barcode analysis provides a rapid readout of cellular signaling pathways in clinical specimens". Nature Communications. 9 (1): 4550. Bibcode:2018NatCo...9.4550G. doi:10.1038/s41467-018-07002-6. ISSN 2041-1723. PMC 6208406. PMID 30382095.
- Lin, Jia-Ren; Izar, Benjamin; Wang, Shu; Yapp, Clarence; Mei, Shaolin; Shah, Parin M; Santagata, Sandro; Sorger, Peter K (2018-07-11). Chakraborty, Arup K; Raj, Arjun; Marr, Carsten; Horváth, Péter (eds.). "Highly multiplexed immunofluorescence imaging of human tissues and tumors using t-CyCIF and conventional optical microscopes". eLife. 7: e31657. doi:10.7554/eLife.31657. ISSN 2050-084X. PMC 6075866. PMID 29993362.
- Knoblich, Juergen A. (February 2008). "Mechanisms of Asymmetric Stem Cell Division". Cell. 132 (4): 583–597. doi:10.1016/j.cell.2008.02.007. PMID 18295577.
- Cohen, A. R. (3 November 2014). "Extracting meaning from biological imaging data". Molecular Biology of the Cell. 25 (22): 3470–3473. doi:10.1091/mbc.E14-04-0946. PMC 4230605. PMID 25368423.