During an election year, we often read news articles that state confidence intervals in terms of proportions or percentages. For example, a poll for a particular presidential candidate might show that the candidate has 40% of the vote, within 3 percentage points. Often, election polls are calculated with 95% confidence. This mean that pollsters are 95% confident that the true proportion of voters who favor the candidate lies between 0.37 and 0.43:
Investors in the stock market are interested in the true proportion of stock values that go up and down each week. Businesses that sell personal computers are interested in the proportion of households (say, in the United States) that own personal computers. Confidence intervals can be calculated for both scenarios.
Although the procedure to find the confidence interval, sample size, error bound, and confidence level for a proportion is similar to that for the population mean, the formulas are different.
Proportion Problems
How do you know if you are dealing with a proportion problem? First, the underlying distribution is binomial (i.e., there is no mention of a mean or average). If
Sometimes the random variable is denoted as
When
If we divide the random variable by
Using algebra to simplify:
The confidence interval has the form
-
$\displaystyle{{ p }^{ ' }=\frac { x }{ n }}$ -
$p'$ is the estimated proportion of successes ($p'$ is a point estimate for$p$ , the true proportion) -
$x$ is the number of successes -
$n$ is the size of the sample
The error bound for a proportion is seen in the formula in:
where
This formula is similar to the error bound formula for a mean, except that the "appropriate standard deviation" is different. For a mean, when the population standard deviation is known, the appropriate standard deviation that we use is
However, in the error bound formula, we use
In the error bound formula, the sample proportions
The confidence interval can only be used if the number of successes
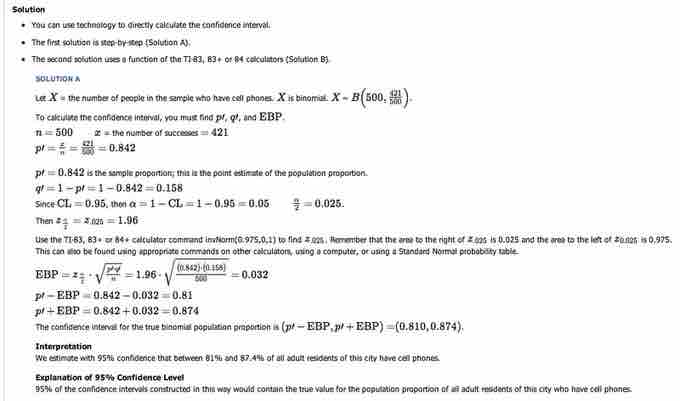
Solution
This image shows the solution to our example.