Cloud feedback
Cloud feedback is a type of climate change feedback that has been difficult to quantify in contemporary climate models. It can affect the magnitude of internally generated climate variability[1][2] or they can affect the magnitude of climate change resulting from external radiative forcings.[3] Cloud representations vary among global climate models, and small changes in cloud cover have a large impact on the climate.[4][5]
.png.webp)
Global warming is expected to change the distribution and type of clouds.[6][7] Seen from below, clouds emit infrared radiation back to the surface, and so exert a warming effect; seen from above, clouds reflect sunlight and emit infrared radiation to space, and so exert a cooling effect.[8] Differences in planetary boundary layer cloud modeling schemes can lead to large differences in derived values of climate sensitivity. A model that decreases boundary layer clouds in response to global warming has a climate sensitivity twice that of a model that does not include this feedback.[9] However, satellite data show that cloud optical thickness actually increases with increasing temperature.[10] Whether the net effect is warming or cooling depends on details such as the type and altitude of the cloud; details that are difficult to represent in climate models.
The closely related effective climate sensitivity has increased substantially in the latest generation of global climate models. Differences in the physical representation of clouds in models drive this enhanced sensitivity relative to the previous generation of models.[11][12][13]
Mechanisms

Global warming is expected to change the distribution and type of clouds. Seen from below, clouds emit infrared radiation back to the surface, and so exert a warming effect; seen from above, clouds reflect sunlight and emit infrared radiation to space, and so exert a cooling effect. Whether the net effect is warming or cooling depends on details such as the type and altitude of the cloud. Low clouds are brighter and optically thicker, while high clouds are optically thin (transparent) in the visible and trap IR. Reduction of low clouds tends to increase incoming solar radiation and therefore have a positive feedback, while a reduction in high clouds (since they mostly just trap IR) would result in a negative feedback. These details were poorly observed before the advent of satellite data and are difficult to represent in climate models.[17] Global climate models were showing a near-zero to moderately strong positive net cloud feedback, but the effective climate sensitivity has increased substantially in the latest generation of global climate models. Differences in the physical representation of clouds in models drive this enhanced climate sensitivity relative to the previous generation of models.[18][19][20]
A 2019 simulation predicts that if greenhouse gases reach three times the current level of atmospheric carbon dioxide that stratocumulus clouds could abruptly disperse, contributing to additional global warming.[21][22]Role as contributor to climate sensitivity
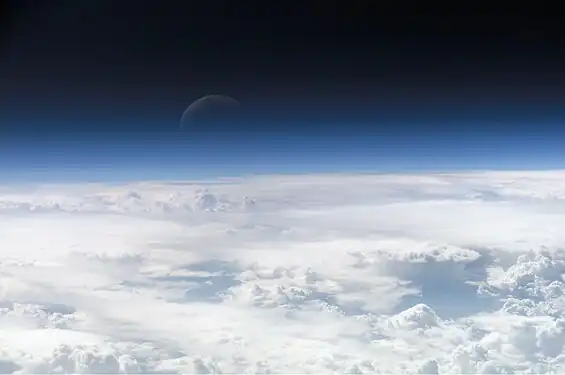
Changes in cloud cover is one of several contributors to climate change and climate sensitivity.
Radiative forcing is one component of climate change. The radiative forcing caused by a doubling of atmospheric CO2 levels (from the pre-industrial 280 ppm) is approximately 3.7 watts per square meter (W/m2). In the absence of feedbacks, the energy imbalance would eventually result in roughly 1 °C (1.8 °F) of global warming. That figure is straightforward to calculate by using the Stefan–Boltzmann law[23][24] and is undisputed.[25]
A further contribution arises from climate feedback, both exacerbating and suppressing.[26][27] The uncertainty in climate sensitivity estimates is entirely from the modelling of feedbacks in the climate system, including water vapour feedback, ice–albedo feedback, cloud feedback, and lapse rate feedback.[25] Suppressing feedbacks tend to counteract warming by increasing the rate at which energy is radiated to space from a warmer planet. Exacerbating feedbacks increase warming; for example, higher temperatures can cause ice to melt, which reduces the ice area and the amount of sunlight the ice reflects, which in turn results in less heat energy being radiated back into space. Climate sensitivity depends on the balance between those feedbacks.[24]Current understanding in climate models
When the IPCC began to produce its IPCC Sixth Assessment Report, many climate models began to show a higher climate sensitivity. The estimates for Equilibrium Climate Sensitivity changed from 3.2 °C to 3.7 °C and the estimates for the Transient climate response from 1.8 °C, to 2.0 °C. That is probably because of better understanding of the role of clouds and aerosols.[28]
In preparation for the 2021 IPCC Sixth Assessment Report, a new generation of climate models have been developed by scientific groups around the world.[29][30] The average estimated climate sensitivity has increased in Coupled Model Intercomparison Project Phase 6 (CMIP6) compared to the previous generation, with values spanning 1.8 to 5.6 °C (3.2 to 10.1 °F) across 27 global climate models and exceeding 4.5 °C (8.1 °F) in 10 of them.[31][32] The cause of the increased equilibrium climate sensitivity (ECS) lies mainly in improved modelling of clouds. Temperature rises are now believed to cause sharper decreases in the number of low clouds, and fewer low clouds means more sunlight is absorbed by the planet and less reflected to space.[31][33][34] Models with the highest ECS values, however, are not consistent with observed warming.[35]
A 2019 simulation predicts that if greenhouse gases reach three times the current level of atmospheric carbon dioxide that stratocumulus clouds could abruptly disperse, contributing to additional global warming.[36]
Relationship with other feedbacks
In addition to how clouds themselves will respond to increased temperatures, other feedbacks affect clouds properties and formation. The amount and vertical distribution of water vapor is closely linked to the formation of clouds. Ice crystals have been shown to largely influence the amount of water vapor.[37] Water vapor in the subtropical upper troposphere has been linked to the convection of water vapor and ice. Changes in subtropical humidity could provide a negative feedback that decreases the amount of water vapor which in turn would act to mediate global climate transitions.[38]
Changes in cloud cover are closely coupled with other feedback, including the water vapor feedback and ice–albedo feedback. Changing climate is expected to alter the relationship between cloud ice and supercooled cloud water, which in turn would influence the microphysics of the cloud which would result in changes in the radiative properties of the cloud. Climate models suggest that a warming will increase fractional cloudiness. The albedo of increased cloudiness cools the climate, resulting in a negative feedback; while the reflection of infrared radiation by clouds warms the climate, resulting in a positive feedback.[39] Increasing temperatures in the polar regions is expected in increase the amount of low-level clouds, whose stratification prevents the convection of moisture to upper levels. This feedback would partially cancel the increased surface warming due to the cloudiness. This negative feedback has less effect than the positive feedback. The upper atmosphere more than cancels negative feedback that causes cooling, and therefore the increase of CO2 is actually exacerbating the positive feedback as more CO2 enters the system.[40]
See also
References
- Brown, Patrick T.; Li, Wenhong; Jiang, Jonathan H.; Su, Hui (2015-12-07). "Unforced Surface Air Temperature Variability and Its Contrasting Relationship with the Anomalous TOA Energy Flux at Local and Global Spatial Scales" (PDF). Journal of Climate. 29 (3): 925–940. Bibcode:2016JCli...29..925B. doi:10.1175/JCLI-D-15-0384.1. ISSN 0894-8755. Archived (PDF) from the original on 2018-07-19.
- Bellomo, Katinka; Clement, Amy; Mauritsen, Thorsten; Rädel, Gaby; Stevens, Bjorn (2014-04-11). "Simulating the Role of Subtropical Stratocumulus Clouds in Driving Pacific Climate Variability". Journal of Climate. 27 (13): 5119–5131. Bibcode:2014JCli...27.5119B. doi:10.1175/JCLI-D-13-00548.1. hdl:11858/00-001M-0000-0014-72C1-F. ISSN 0894-8755. S2CID 33019270.
- Stephens, Graeme L. (2005-01-01). "Cloud Feedbacks in the Climate System: A Critical Review". Journal of Climate. 18 (2): 237–273. Bibcode:2005JCli...18..237S. CiteSeerX 10.1.1.130.1415. doi:10.1175/JCLI-3243.1. ISSN 0894-8755. S2CID 16122908.
- Cess, R. D.; et al. (1990). "Intercomparison and Interpretation of Climate Feedback Processes in 19 Atmospheric General Circulation Models" (PDF). J. Geophys. Res. 95 (D10): 16, 601–16, 615. Bibcode:1990JGR....9516601C. doi:10.1029/jd095id10p16601. Archived from the original (PDF) on 2018-07-22. Retrieved 2017-10-27.
- Stocker, T.F.; et al. (2001). "Physical climate processes and feedbacks". In J.T. Houghton; et al. (eds.). Climate Change 2001: The Scientific Basis, Contributions of Working Group I to the Third Assessment Report of the Intergovernmental Panel on Climate Change. Cambridge, U.K.: Cambridge University Press.
- GREENFIELDBOYCE, Nell (July 11, 2016). "Climate Change May Already Be Shifting Clouds Toward The Poles". NPR. Retrieved July 11, 2016.
- "How climate change is altering Earth's cloud cover". The Christian Science Monitor. July 12, 2016. Retrieved July 14, 2016.
- Hartmann, D.L.; M.E. Ockert-Bell & M.L. Michelsen (1992). "The Effect of Cloud Type on Earth's Energy Balance: Global Analysis". J. Climate. 5 (11): 1281–1304. Bibcode:1992JCli....5.1281H. doi:10.1175/1520-0442(1992)005<1281:TEOCTO>2.0.CO;2.
- National Research Council (2004). Understanding Climate Change Feedbacks. Panel on Climate Change Feedbacks, Climate Research Committee. National Academies Press. doi:10.17226/10850. ISBN 978-0-309-09072-8.
- Tselioudis, G.; W.B. Rossow & D. Rind (1992). "Global Patterns of Cloud Optical Thickness Variation with Temperature". J. Climate. 5 (12): 1484–1495. Bibcode:1992JCli....5.1484T. doi:10.1175/1520-0442(1992)005<1484:GPOCOT>2.0.CO;2.
- Zelinka, Mark D.; Myers, Timothy A.; McCoy, Daniel T.; Po‐Chedley, Stephen; Caldwell, Peter M.; Ceppi, Paulo; Klein, Stephen A.; Taylor, Karl E. (2020). "Causes of Higher Climate Sensitivity in CMIP6 Models". Geophysical Research Letters. 47 (1): e2019GL085782. Bibcode:2020GeoRL..4785782Z. doi:10.1029/2019GL085782. hdl:10044/1/76038. ISSN 1944-8007.
- Watts, Jonathan (2020-06-13). "Climate worst-case scenarios may not go far enough, cloud data shows". the Guardian. Retrieved 2020-06-19.
- Palmer, Tim (2020-05-26). "Short-term tests validate long-term estimates of climate change". Nature. 582 (7811): 185–186. Bibcode:2020Natur.582..185P. doi:10.1038/d41586-020-01484-5. PMID 32457461.
- "The Study of Earth as an Integrated System". nasa.gov. NASA. 2016. Archived from the original on November 2, 2016.
- Fig. TS.17, Technical Summary, Sixth Assessment Report (AR6), Working Group I, IPCC, 2021, p. 96. Archived from the original on July 21, 2022.
- Stocker, Thomas F.; Dahe, Qin; Plattner, Gian-Kaksper (2013). IPCC AR5 WG1. Technical Summary (PDF). Archived (PDF) from the original on 16 July 2023. See esp. TFE.6: Climate Sensitivity and Feedbacks at p. 82.
- Soden, B. J.; Held, I. M. (2006). "An Assessment of Climate Feedbacks in Coupled Ocean–Atmosphere Models". Journal of Climate. 19 (14): 3354. Bibcode:2006JCli...19.3354S. doi:10.1175/JCLI3799.1.
Interestingly, the true feedback is consistently weaker than the constant relative humidity value, implying a small but robust reduction in relative humidity in all models on average clouds appear to provide a positive feedback in all models
- Zelinka, Mark D.; Myers, Timothy A.; McCoy, Daniel T.; Po‐Chedley, Stephen; Caldwell, Peter M.; Ceppi, Paulo; Klein, Stephen A.; Taylor, Karl E. (2020). "Causes of Higher Climate Sensitivity in CMIP6 Models". Geophysical Research Letters. 47 (1): e2019GL085782. Bibcode:2020GeoRL..4785782Z. doi:10.1029/2019GL085782. ISSN 1944-8007.
- Watts, Jonathan (2020-06-13). "Climate worst-case scenarios may not go far enough, cloud data shows". The Guardian. ISSN 0261-3077. Retrieved 2020-06-19.
- Palmer, Tim (2020-05-26). "Short-term tests validate long-term estimates of climate change". Nature. 582 (7811): 185–186. Bibcode:2020Natur.582..185P. doi:10.1038/d41586-020-01484-5. PMID 32457461.
- Pressel, Kyle G.; Kaul, Colleen M.; Schneider, Tapio (March 2019). "Possible climate transitions from breakup of stratocumulus decks under greenhouse warming" (PDF). Nature Geoscience. 12 (3): 163–167. Bibcode:2019NatGe..12..163S. doi:10.1038/s41561-019-0310-1. ISSN 1752-0908. S2CID 134307699.
- Kemp, Luke; Xu, Chi; Depledge, Joanna; Ebi, Kristie L.; Gibbins, Goodwin; Kohler, Timothy A.; Rockström, Johan; Scheffer, Marten; Schellnhuber, Hans Joachim; Steffen, Will; Lenton, Timothy M. (2022-08-23). "Climate Endgame: Exploring catastrophic climate change scenarios". Proceedings of the National Academy of Sciences. 119 (34): e2108146119. Bibcode:2022PNAS..11908146K. doi:10.1073/pnas.2108146119. ISSN 0027-8424. PMC 9407216. PMID 35914185.
- The calculation is as follows. In equilibrium, the energy of incoming and outgoing radiation have to balance. The outgoing radiation is given by the Stefan–Boltzmann law: . When incoming radiation increases, the outgoing radiation and therefore temperature must increase as well. The temperature rise directly caused by the additional radiative forcing, because of the doubling of CO2 is then given by
- .
- Roe G (2009). "Feedbacks, Timescales, and Seeing Red". Annual Review of Earth and Planetary Sciences. 37 (1): 93–115. Bibcode:2009AREPS..37...93R. doi:10.1146/annurev.earth.061008.134734. S2CID 66109238.
- Rahmstorf S (2008). "Anthropogenic Climate Change: Revisiting the Facts" (PDF). In Zedillo E (ed.). Global Warming: Looking Beyond Kyoto. Brookings Institution Press. pp. 34–53. Archived (PDF) from the original on 14 July 2019. Retrieved 14 August 2008.
- Lenton TM, Rockström J, Gaffney O, Rahmstorf S, Richardson K, Steffen W, Schellnhuber HJ (November 2019). "Climate tipping points - too risky to bet against". Nature. 575 (7784): 592–595. Bibcode:2019Natur.575..592L. doi:10.1038/d41586-019-03595-0. PMID 31776487.
- Armstrong McKay, David I.; Staal, Arie; Abrams, Jesse F.; Winkelmann, Ricarda; Sakschewski, Boris; Loriani, Sina; Fetzer, Ingo; Cornell, Sarah E.; Rockström, Johan; Lenton, Timothy M. (2022-09-09). "Exceeding 1.5°C global warming could trigger multiple climate tipping points". Science. 377 (6611): eabn7950. doi:10.1126/science.abn7950. hdl:10871/131584. ISSN 0036-8075. PMID 36074831. S2CID 252161375.
- "Increased warming in latest generation of climate models likely caused by clouds: New representations of clouds are making models more sensitive to carbon dioxide". Science Daily. 24 June 2020. Archived from the original on 26 June 2020. Retrieved 26 June 2020.
- "The CMIP6 landscape (Editorial)". Nature Climate Change. 9 (10): 727. 2019-09-25. Bibcode:2019NatCC...9..727.. doi:10.1038/s41558-019-0599-1. ISSN 1758-6798.
- "New climate models suggest Paris goals may be out of reach". France 24. 2020-01-14. Archived from the original on 14 January 2020. Retrieved 2020-01-18.
- Zelinka MD, Myers TA, McCoy DT, Po-Chedley S, Caldwell PM, Ceppi P, Klein SA, Taylor KE (2020). "Causes of Higher Climate Sensitivity in CMIP6 Models". Geophysical Research Letters. 47 (1): e2019GL085782. Bibcode:2020GeoRL..4785782Z. doi:10.1029/2019GL085782. ISSN 1944-8007.
- "International analysis narrows range of climate's sensitivity to CO2". UNSW Newsroom. 2020-07-23. Archived from the original on 23 July 2020. Retrieved 2020-07-23.
- Palmer, Tim (2020-05-26). "Short-term tests validate long-term estimates of climate change". Nature. 582 (7811): 185–186. Bibcode:2020Natur.582..185P. doi:10.1038/d41586-020-01484-5. PMID 32457461.
- Watts, Jonathan (2020-06-13). "Climate worst-case scenarios may not go far enough, cloud data shows". The Guardian. ISSN 0261-3077. Archived from the original on 19 June 2020. Retrieved 2020-06-19.
- Bender M (2020-02-07). "Climate Change Predictions Have Suddenly Gone Catastrophic. This Is Why". Vice. Archived from the original on 10 February 2020. Retrieved 2020-02-09.
- Pressel, Kyle G.; Kaul, Colleen M.; Schneider, Tapio (March 2019). "Possible climate transitions from breakup of stratocumulus decks under greenhouse warming". Nature Geoscience. 12 (3): 163–167. Bibcode:2019NatGe..12..163S. doi:10.1038/s41561-019-0310-1. ISSN 1752-0908. S2CID 134307699.
- Donner, L. J.; C. J. Seman; B. J. Soden; R. S. Hemler; J. C. Warren; J. Ström & K.-N. Liou (1997). "Large-scale ice clouds in the GFDL SKYHI general circulation model". J. Geophys. Res. 102 (D18): 21, 745–21, 768. Bibcode:1997JGR...10221745D. doi:10.1029/97JD01488.
- Pierrehumbert, R. T.; R. Roca (1998). "Evidence for Control of Atlantic Subtropical Humidity by Large Scale Advection" (PDF). Geophys. Res. Lett. 25 (24): 4537–4540. Bibcode:1998GeoRL..25.4537P. doi:10.1029/1998GL900203. Archived (PDF) from the original on 2003-06-08.
- Fowler, L.D.; D.A. Randall (1996). "Liquid and Ice Cloud Microphysics in the CSU General Circulation Model. Part III: Sensitivity to Modeling Assumptions". J. Climate. 9 (3): 561–586. Bibcode:1996JCli....9..561F. doi:10.1175/1520-0442(1996)009<0561:LAICMI>2.0.CO;2.
- Wetherald, R.; S. Manabe (1988). "Cloud Feedback Processes in a General Circulation Model". J. Atmos. Sci. 45 (8): 1397–1416. Bibcode:1988JAtS...45.1397W. doi:10.1175/1520-0469(1988)045<1397:CFPIAG>2.0.CO;2.