High-throughput screening
High-throughput screening (HTS) is a method for scientific experimentation especially used in drug discovery and relevant to the fields of biology, materials science[1] and chemistry.[2][3] Using robotics, data processing/control software, liquid handling devices, and sensitive detectors, high-throughput screening allows a researcher to quickly conduct millions of chemical, genetic, or pharmacological tests. Through this process one can quickly recognize active compounds, antibodies, or genes that modulate a particular biomolecular pathway. The results of these experiments provide starting points for drug design and for understanding the noninteraction or role of a particular location.

Assay plate preparation
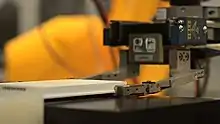
The key labware or testing vessel of HTS is the microtiter plate, which is a small container, usually disposable and made of plastic, that features a grid of small, open divots called wells. In general, microplates for HTS have either 96, 192, 384, 1536, 3456 or 6144 wells. These are all multiples of 96, reflecting the original 96-well microplate with spaced wells of 8 x 12 with 9 mm spacing. Most of the wells contain test items, depending on the nature of the experiment. These could be different chemical compounds dissolved e.g. in an aqueous solution of dimethyl sulfoxide (DMSO). The wells could also contain cells or enzymes of some type. (The other wells may be empty or contain pure solvent or untreated samples, intended for use as experimental controls.)
A screening facility typically holds a library of stock plates, whose contents are carefully catalogued, and each of which may have been created by the lab or obtained from a commercial source. These stock plates themselves are not directly used in experiments; instead, separate assay plates are created as needed. An assay plate is simply a copy of a stock plate, created by pipetting a small amount of liquid (often measured in nanoliters) from the wells of a stock plate to the corresponding wells of a completely empty plate.
Reaction observation
To prepare for an assay, the researcher fills each well of the plate with some biological entity that they wish to conduct the experiment upon, such as a protein, cells, or an animal embryo. After some incubation time has passed to allow the biological matter to absorb, bind to, or otherwise react (or fail to react) with the compounds in the wells, measurements are taken across all the plate's wells, either manually or by a machine. Manual measurements are often necessary when the researcher is using microscopy to (for example) seek changes or defects in embryonic development caused by the wells' compounds, looking for effects that a computer could not easily determine by itself. Otherwise, a specialized automated analysis machine can run a number of experiments on the wells (such as shining polarized light on them and measuring reflectivity, which can be an indication of protein binding). In this case, the machine outputs the result of each experiment as a grid of numeric values, with each number mapping to the value obtained from a single well. A high-capacity analysis machine can measure dozens of plates in the space of a few minutes like this, generating thousands of experimental datapoints very quickly.
Depending on the results of this first assay, the researcher can perform follow up assays within the same screen by "cherrypicking" liquid from the source wells that gave interesting results (known as "hits") into new assay plates, and then re-running the experiment to collect further data on this narrowed set, confirming and refining observations.
Automation systems

Automation is an essential element in HTS's usefulness. Typically, an integrated robot system consisting of one or more robots transports assay-microplates from station to station for sample and reagent addition, mixing, incubation, and finally readout or detection. An HTS system can usually prepare, incubate, and analyze many plates simultaneously, further speeding the data-collection process. HTS robots that can test up to 100,000 compounds per day currently exist.[4][5] Automatic colony pickers pick thousands of microbial colonies for high throughput genetic screening.[6] The term uHTS or ultra-high-throughput screening refers (circa 2008) to screening in excess of 100,000 compounds per day.[7]
Experimental design and data analysis
With the ability of rapid screening of diverse compounds (such as small molecules or siRNAs) to identify active compounds, HTS has led to an explosion in the rate of data generated in recent years .[8] Consequently, one of the most fundamental challenges in HTS experiments is to glean biochemical significance from mounds of data, which relies on the development and adoption of appropriate experimental designs and analytic methods for both quality control and hit selection .[9] HTS research is one of the fields that have a feature described by John Blume, Chief Science Officer for Applied Proteomics, Inc., as follows: Soon, if a scientist does not understand some statistics or rudimentary data-handling technologies, he or she may not be considered to be a true molecular biologist and, thus, will simply become "a dinosaur."[10]
Quality control
High-quality HTS assays are critical in HTS experiments. The development of high-quality HTS assays requires the integration of both experimental and computational approaches for quality control (QC). Three important means of QC are (i) good plate design, (ii) the selection of effective positive and negative chemical/biological controls, and (iii) the development of effective QC metrics to measure the degree of differentiation so that assays with inferior data quality can be identified. [11] A good plate design helps to identify systematic errors (especially those linked with well position) and determine what normalization should be used to remove/reduce the impact of systematic errors on both QC and hit selection.[9]
Effective analytic QC methods serve as a gatekeeper for excellent quality assays. In a typical HTS experiment, a clear distinction between a positive control and a negative reference such as a negative control is an index for good quality. Many quality-assessment measures have been proposed to measure the degree of differentiation between a positive control and a negative reference. Signal-to-background ratio, signal-to-noise ratio, signal window, assay variability ratio, and Z-factor have been adopted to evaluate data quality. [9] [12] Strictly standardized mean difference (SSMD) has recently been proposed for assessing data quality in HTS assays. [13] [14]
Hit selection
A compound with a desired size of effects in an HTS is called a hit. The process of selecting hits is called hit selection. The analytic methods for hit selection in screens without replicates (usually in primary screens) differ from those with replicates (usually in confirmatory screens). For example, the z-score method is suitable for screens without replicates whereas the t-statistic is suitable for screens with replicates. The calculation of SSMD for screens without replicates also differs from that for screens with replicates .[9]
For hit selection in primary screens without replicates, the easily interpretable ones are average fold change, mean difference, percent inhibition, and percent activity. However, they do not capture data variability effectively. The z-score method or SSMD, which can capture data variability based on an assumption that every compound has the same variability as a negative reference in the screens. [15][16] However, outliers are common in HTS experiments, and methods such as z-score are sensitive to outliers and can be problematic. As a consequence, robust methods such as the z*-score method, SSMD*, B-score method, and quantile-based method have been proposed and adopted for hit selection.[5] [9] [17] [18]
In a screen with replicates, we can directly estimate variability for each compound; as a consequence, we should use SSMD or t-statistic that does not rely on the strong assumption that the z-score and z*-score rely on. One issue with the use of t-statistic and associated p-values is that they are affected by both sample size and effect size.[19] They come from testing for no mean difference, and thus are not designed to measure the size of compound effects. For hit selection, the major interest is the size of effect in a tested compound. SSMD directly assesses the size of effects.[20] SSMD has also been shown to be better than other commonly used effect sizes.[21] The population value of SSMD is comparable across experiments and, thus, we can use the same cutoff for the population value of SSMD to measure the size of compound effects .[22]
Techniques for increased throughput and efficiency
Unique distributions of compounds across one or many plates can be employed either to increase the number of assays per plate or to reduce the variance of assay results, or both. The simplifying assumption made in this approach is that any N compounds in the same well will not typically interact with each other, or the assay target, in a manner that fundamentally changes the ability of the assay to detect true hits.
For example, imagine a plate wherein compound A is in wells 1-2-3, compound B is in wells 2-3-4, and compound C is in wells 3-4-5. In an assay of this plate against a given target, a hit in wells 2, 3, and 4 would indicate that compound B is the most likely agent, while also providing three measurements of compound B's efficacy against the specified target. Commercial applications of this approach involve combinations in which no two compounds ever share more than one well, to reduce the (second-order) possibility of interference between pairs of compounds being screened.
Recent advances
Automation and low volume assay formats were leveraged by scientists at the NIH Chemical Genomics Center (NCGC) to develop quantitative HTS (qHTS), a paradigm to pharmacologically profile large chemical libraries through the generation of full concentration-response relationships for each compound. With accompanying curve fitting and cheminformatics software qHTS data yields half maximal effective concentration (EC50), maximal response, Hill coefficient (nH) for the entire library enabling the assessment of nascent structure activity relationships (SAR).[23]
In March 2010, research was published demonstrating an HTS process allowing 1,000 times faster screening (100 million reactions in 10 hours) at 1-millionth the cost (using 10−7 times the reagent volume) than conventional techniques using drop-based microfluidics.[23] Drops of fluid separated by oil replace microplate wells and allow analysis and hit sorting while reagents are flowing through channels.
In 2010, researchers developed a silicon sheet of lenses that can be placed over microfluidic arrays to allow the fluorescence measurement of 64 different output channels simultaneously with a single camera.[24] This process can analyze 200,000 drops per second.
In 2013, researchers have disclosed an approach with small molecules from plants. In general, it is essential to provide high-quality proof-of-concept validations early in the drug discovery process. Here technologies that enable the identification of potent, selective, and bioavailable chemical probes are of crucial interest, even if the resulting compounds require further optimization for development into a pharmaceutical product. Nuclear receptor RORα, a protein that has been targeted for more than a decade to identify potent and bioavailable agonists, was used as an example of a very challenging drug target. Hits are confirmed at the screening step due to the bell-shaped curve. This method is very similar to the quantitative HTS method (screening and hit confirmation at the same time), except that using this approach greatly decreases the data point number and can screen easily more than 100.000 biological relevant compounds.[25]
Whereby traditional HTS drug discovery uses purified proteins or intact cells, recent development of the technology is associated with the use of intact living organisms, like the nematode Caenorhabditis elegans and zebrafish (Danio rerio).[26]
In 2016-2018 plate manufacturers began producing specialized chemistry to allow for mass production of ultra-low adherent cell repellent surfaces which facilitated the rapid development of HTS amenable assays to address cancer drug discovery in 3D tissues such as organoids and spheroids; a more physiologically relevant format.[27][28][29]
Increasing use of HTS in academia for biomedical research
HTS is a relatively recent innovation, made feasible largely through modern advances in robotics and high-speed computer technology. It still takes a highly specialized and expensive screening lab to run an HTS operation, so in many cases a small- to moderate-size research institution will use the services of an existing HTS facility rather than set up one for itself.
There is a trend in academia for universities to be their own drug discovery enterprise.[30] These facilities, which normally are found only in industry, are now increasingly found at universities as well. UCLA, for example, features an open access HTS laboratory Molecular Screening Shared Resources (MSSR, UCLA), which can screen more than 100,000 compounds a day on a routine basis. The open access policy ensures that researchers from all over the world can take advantage of this facility without lengthy intellectual property negotiations. With a compound library of over 200,000 small molecules, the MSSR has one of the largest compound deck of all universities on the west coast. Also, the MSSR features full functional genomics capabilities (genome wide siRNA, shRNA, cDNA and CRISPR) which are complementary to small molecule efforts: Functional genomics leverages HTS capabilities to execute genome wide screens which examine the function of each gene in the context of interest by either knocking each gene out or overexpressing it. Parallel access to high-throughput small molecule screen and a genome wide screen enables researchers to perform target identification and validation for given disease or the mode of action determination on a small molecule. The most accurate results can be obtained by use of "arrayed" functional genomics libraries, i.e. each library contains a single construct such as a single siRNA or cDNA. Functional genomics is typically paired with high content screening using e.g. epifluorescent microscopy or laser scanning cytometry.
The University of Illinois also has a facility for HTS, as does the University of Minnesota. The Life Sciences Institute at the University of Michigan houses the HTS facility in the Center for Chemical Genomics. Columbia University has an HTS shared resource facility with ~300,000 diverse small molecules and ~10,000 known bioactive compounds available for biochemical, cell-based and NGS-based screening. The Rockefeller University has an open-access HTS Resource Center HTSRC (The Rockefeller University, HTSRC), which offers a library of over 380,000 compounds. Northwestern University's High Throughput Analysis Laboratory supports target identification, validation, assay development, and compound screening. The non-profit Sanford Burnham Prebys Medical Discovery Institute also has a long-standing HTS facility in the Conrad Prebys Center for Chemical Genomics which was part of the MLPCN. The non-profit Scripps Research Molecular Screening Center (SRMSC)[31] continues to serve academia across institutes post-MLPCN era. The SRMSC uHTS facility maintains one of the largest library collections in academia, presently at well-over 665,000 small molecule entities, and routinely screens the full collection or sub-libraries in support of multi-PI grant initiatives.
In the United States, the National Institutes of Health or NIH has created a nationwide consortium of small-molecule screening centers to produce innovative chemical tools for use in biological research. The Molecular Libraries Probe Production Centers Network, or MLPCN, performs HTS on assays provided by the research community, against a large library of small molecules maintained in a central molecule repository.
See also
References
- Zhao, Yicheng; Heumueller, Thomas; Zhang, Jiyun; Luo, Junsheng; Kasian, Olga; Langner, Stefan; Kupfer, Christian; Liu, Bowen; Zhong, Yu; Elia, Jack; Osvet, Andres; Wu, Jianchang; Liu, Chao; Wan, Zhongquan; Jia, Chunyang; Li, Ning; Hauch, Jens; Brabec, Christoph J. (16 December 2021). "A bilayer conducting polymer structure for planar perovskite solar cells with over 1,400 hours operational stability at elevated temperatures". Nature Energy. 7 (2): 144–152. Bibcode:2022NatEn...7..144Z. doi:10.1038/s41560-021-00953-z. ISSN 2058-7546. S2CID 245285868.
- Inglese J and Auld DS. (2009) Application of High Throughput Screening (HTS) Techniques: Applications in Chemical Biology in Wiley Encyclopedia of Chemical Biology (Wiley & Sons, Inc., Hoboken, NJ) Vol 2, pp 260–274 doi/10.1002/9780470048672.wecb223.
- Macarron, R.; Banks, M.N.; Bojanic, D.; Burns, D.J.; Cirovic, D.A.; Garyantes, T.; Green, D.V.; Hertzberg, R.P.; Janzen, W.P.; Paslay, J.W.; Schopfer, U.; Sittampalam, G.S. (2011). "Impact of high-throughput screening in biomedical research". Nat Rev Drug Discov. 10 (3): 188–195. doi:10.1038/nrd3368. PMID 21358738. S2CID 205477370.
- Hann MM, Oprea TI (June 2004). "Pursuing the leadlikeness concept in pharmaceutical research". Curr Opin Chem Biol. 8 (3): 255–63. doi:10.1016/j.cbpa.2004.04.003. PMID 15183323.
- Caraus, I.; Alsuwailem, A. A.; Nadon, R.; Makarenkov, V. (2015-11-01). "Detecting and overcoming systematic bias in high-throughput screening technologies: a comprehensive review of practical issues and methodological solutions". Briefings in Bioinformatics. 16 (6): 974–986. doi:10.1093/bib/bbv004. ISSN 1467-5463. PMID 25750417.
- Heddle, C.; Mazaleyrat, S. L. (2007). "Development of a screening platform for directed evolution using the reef coral fluorescent protein ZsGreen as a solubility reporter". Protein Engineering Design and Selection. 20 (7): 327–337. doi:10.1093/protein/gzm024. ISSN 1741-0126. PMID 17584755.
- Michael, Sam; Auld, Douglas; Klumpp, Carleen; Jadhav, Ajit; Zheng, Wei; Thorne, Natasha; Austin, Christopher P.; Inglese, James; Simeonov, Anton (2008). "A Robotic Platform for Quantitative High-Throughput Screening". ASSAY and Drug Development Technologies. 6 (5): 637–657. doi:10.1089/adt.2008.150. ISSN 1540-658X. PMC 2651822. PMID 19035846.
- Howe D, Costanzo M, Fey P, Gojobori T, Hannick L, Hide W, Hill DP, Kania R, Schaeffer M, Pierre SS, Twigger S, White O, Rhee SY (2008). "Big data: The future of biocuration". Nature. 455 (7209): 47–50. Bibcode:2008Natur.455...47H. doi:10.1038/455047a. PMC 2819144. PMID 18769432.
- Zhang XHD (2011). Optimal High-Throughput Screening: Practical Experimental Design and Data Analysis for Genome-scale RNAi Research. Cambridge University Press. ISBN 978-0-521-73444-8.
- Eisenstein M (2006). "Quality control". Nature. 442 (7106): 1067–70. Bibcode:2006Natur.442.1067E. doi:10.1038/4421067a. PMID 16943838.
- Zhang XH, Espeseth AS, Johnson EN, Chin J, Gates A, Mitnaul LJ, Marine SD, Tian J, Stec EM, Kunapuli P, Holder DJ, Heyse JF, Strulocivi B, Ferrer M (2008). "Integrating experimental and analytic approaches to improve data quality in genome-scale RNAi screens". Journal of Biomolecular Screening. 13 (5): 378–89. doi:10.1177/1087057108317145. PMID 18480473. S2CID 22679273.
- Zhang JH, Chung TD, Oldenburg KR (1999). "A simple statistical parameter for use in evaluation and validation of high throughput screening assays". Journal of Biomolecular Screening. 4 (2): 67–73. doi:10.1177/108705719900400206. PMID 10838414. S2CID 36577200.
- Zhang, XHD (2007). "A pair of new statistical parameters for quality control in RNA interference high-throughput screening assays". Genomics. 89 (4): 552–61. doi:10.1016/j.ygeno.2006.12.014. PMID 17276655.
- Zhang XHD (2008). "Novel analytic criteria and effective plate designs for quality control in genome-scale RNAi screens". Journal of Biomolecular Screening. 13 (5): 363–77. doi:10.1177/1087057108317062. PMID 18567841. S2CID 12688742.
- Zhang XHD (2007). "A new method with flexible and balanced control of false negatives and false positives for hit selection in RNA interference high-throughput screening assays". Journal of Biomolecular Screening. 12 (5): 645–55. doi:10.1177/1087057107300645. PMID 17517904.
- Zhang XH, Ferrer M, Espeseth AS, Marine SD, Stec EM, Crackower MA, Holder DJ, Heyse JF, Strulovici B (2007). "The use of strictly standardized mean difference for hit selection in primary RNA interference high-throughput screening experiments". Journal of Biomolecular Screening. 12 (4): 645–55. doi:10.1177/1087057107300646. PMID 17435171. S2CID 7542230.
- Zhang XH, Yang XC, Chung N, Gates A, Stec E, Kunapuli P, Holder DJ, Ferrer M, Espeseth AS (2006). "Robust statistical methods for hit selection in RNA interference high-throughput screening experiments". Pharmacogenomics. 7 (3): 299–09. doi:10.2217/14622416.7.3.299. PMID 16610941.
- Brideau C, Gunter G, Pikounis B, Liaw A (2003). "Improved statistical methods for hit selection in high-throughput screening". Journal of Biomolecular Screening. 8 (6): 634–47. doi:10.1177/1087057103258285. PMID 14711389.
- Cohen J (1994). "The Earth Is Round (P-Less-Than.05)". American Psychologist. 49 (12): 997–1003. doi:10.1037/0003-066X.49.12.997. ISSN 0003-066X.
- Zhang XHD (2009). "A method for effectively comparing gene effects in multiple conditions in RNAi and expression-profiling research". Pharmacogenomics. 10 (3): 345–58. doi:10.2217/14622416.10.3.345. PMID 20397965.
- Zhang XHD (2010). "Strictly standardized mean difference, standardized mean difference and classical t-test for the comparison of two groups". Statistics in Biopharmaceutical Research. 2 (2): 292–99. doi:10.1198/sbr.2009.0074. S2CID 119825625.
- Zhang XHD (2010). "Assessing the size of gene or RNAi effects in multifactor high-throughput experiments". Pharmacogenomics. 11 (2): 199–213. doi:10.2217/PGS.09.136. PMID 20136359.
- Inglese, J.; Auld, D.S.; Jadhav, A.; Johnson, R.L.; Simeonov, A.; Yasgar, A.; Zheng, W.; Austin, C.P. (2006). "Quantitative High-Throughput Screening (qHTS): A Titration-based Approach that Efficiently Identifies Biological Activities in Large Chemical Libraries". Proc. Natl. Acad. Sci. USA. 103 (31): 11473–11478. Bibcode:2006PNAS..10311473I. doi:10.1073/pnas.0604348103. PMC 1518803. PMID 16864780.
- Schonbrun, E; Abate, A. R; Steinvurzel, P. E; Weitz, D. A; Crozier, K. B (2010). "High-throughput fluorescence detection using an integrated zone-plate array". Lab on a Chip. Royal Society of Chemistry. 10 (7): 852–856. CiteSeerX 10.1.1.662.8909. doi:10.1039/b923554j. PMID 20300671.
- "Marriage of microfluidics and optics could advance lab-on-a-chip devices". ScienceDaily (Press release). February 22, 2010.
- Helleboid S, Haug C, Lamottke K, et al. The Identification of Naturally Occurring Neoruscogenin as a Bioavailable, Potent, and High-Affinity Agonist of the Nuclear Receptor RORα (NR1F1). Journal of Biomolecular Screening. 2014;19(3):399-406. https://doi.org/10.1177/1087057113497095.
- Atanasov AG, Waltenberger B, Pferschy-Wenzig EM, Linder T, Wawrosch C, Uhrin P, Temml V, Wang L, Schwaiger S, Heiss EH, Rollinger JM, Schuster D, Breuss JM, Bochkov V, Mihovilovic MD, Kopp B, Bauer R, Dirsch VM, Stuppner H (2015). "Discovery and resupply of pharmacologically active plant-derived natural products: A review". Biotechnol. Adv. 33 (8): 1582–614. doi:10.1016/j.biotechadv.2015.08.001. PMC 4748402. PMID 26281720.
- Kota, S.; Hou, S.; Guerrant, W.; Madoux, F.; Troutman, S.; Fernandez-Vega, V.; Alekseeva, N.; Madala, N.; Scampavia, L.; Kissil, J.; Spicer, TP. (10 May 2018). "A novel three-dimensional high-throughput screening approach identifies inducers of a mutant KRAS selective lethal phenotype". Oncogene. 37 (32): 4372–4384. doi:10.1038/s41388-018-0257-5. PMC 6138545. PMID 29743592.
- Hou, S.; Tiriac, H.; Sridharan, BP.; Scampavia, L.; Madoux, F.; Seldin, J.; Souza, GR.; Watson, D.; Tuveson, D.; Spicer, TP. (July 2018). "Advanced Development of Primary Pancreatic Organoid Tumor Models for High-Throughput Phenotypic Drug Screening". SLAS Discovery. 23 (6): 574–584. doi:10.1177/2472555218766842. PMC 6013403. PMID 29673279.
- Madoux, F.; Tanner, A.; Vessels, M.; Willetts, L.; Hou, S.; Scampavia, L.; Spicer, TP. (June 2017). "A 1536-Well 3D Viability Assay to Assess the Cytotoxic Effect of Drugs on Spheroids". SLAS Discovery. 22 (5): 516–524. doi:10.1177/2472555216686308. PMID 28346088.
- Dove, Alan (2007). "High-throughput screening goes to school". Nature Methods. 4 (6): 523–532. doi:10.1038/nmeth0607-523. ISSN 1548-7091. S2CID 28059031.
- Baillargeon P, Fernandez-Vega V, Sridharan BP, Brown S, Griffin PR, Rosen H, et al. (2019). "The Scripps Molecular Screening Center and Translational Research Institute". SLAS Discov. 24 (3): 386–397. doi:10.1177/2472555218820809. PMC 7724958. PMID 30682260. S2CID 59274228.
Further reading
- Lin, Yibin (2018). "What's happened over the last five years with high-throughput protein crystallization screening?". Expert Opinion on Drug Discovery. 13 (8): 691–695. doi:10.1080/17460441.2018.1465924. ISSN 1746-0441. PMID 29676184.
- Staff (2008-08-01). "High-Throughput Screening Challenges". Genetic Engineering & Biotechnology News. Drug Discovery Rountable Discussion. Vol. 28, no. 14. Mary Ann Liebert. pp. 26–27. ISSN 1935-472X. Retrieved 2008-10-01.
- Zhang XHD (2011) "Optimal High-Throughput Screening: Practical Experimental Design and Data Analysis for Genome-scale RNAi Research, Cambridge University Press"
- Florian, Amy; Lepensky, C; Kwon, O; Sklar, L; Haynes, M (201). "Flow cytometry enbles a high-throughput homogenous fluorecent antibody-binding assay for cytotoxic T cell lytic granule exocytosis". Journal of Biomolecular Screening. 4 (18): 420–429. doi:10.1177/1087057112466697. PMC 4043149. PMID 23160568. Flow cytometry enables a high-throughput homogeneous fluorescent antibody-binding assay for cytotoxic T cell lytic granule exocytosis
- Florian, Amy E.; Lepensky, Christopher K.; Kwon, Ohyun; Haynes, Mark K.; Sklar, Larry A.; Zweifach, Adam (2013). "Flow Cytometry Enables a High-Throughput Homogeneous Fluorescent Antibody-Binding Assay for Cytotoxic T Cell Lytic Granule Exocytosis". Journal of Biomolecular Screening. 18 (4): 420–429. doi:10.1177/1087057112466697. ISSN 1087-0571. PMC 4043149. PMID 23160568.
External links
- Open Screening Environment
- Setting up High-Throughput Screening Laboratory (Koppal, Lab Manager Magazine)
- Assay Guidance Manual (NIH, NCGC)
- OncoSignature High Throughput Screening