Univariate (statistics)
Univariate is a term commonly used in statistics to describe a type of data which consists of observations on only a single characteristic or attribute. A simple example of univariate data would be the salaries of workers in industry.[1] Like all the other data, univariate data can be visualized using graphs, images or other analysis tools after the data is measured, collected, reported, and analyzed.[2]
Univariate data types
Some univariate data consists of numbers (such as the height of 65 inches or the weight of 100 pounds), while others are nonnumerical (such as eye colors of brown or blue). Generally, the terms categorical univariate data and numerical univariate data are used to distinguish between these types.
Categorical univariate data
Categorical univariate data consists of non-numerical observations that may be placed in categories. It includes labels or names used to identify an attribute of each element. Categorical univariate data usually use either nominal or ordinal scale of measurement.[3]
Numerical univariate data
Numerical univariate data consists of observations that are numbers. They are obtained using either interval or ratio scale of measurement. This type of univariate data can be classified even further into two subcategories: discrete and continuous.[4] A numerical univariate data is discrete if the set of all possible values is finite or countably infinite. Discrete univariate data are usually associated with counting (such as the number of books read by a person). A numerical univariate data is continuous if the set of all possible values is an interval of numbers. Continuous univariate data are usually associated with measuring (such as the weights of people).
Data analysis and applications
Univariate analysis is the simplest form of analyzing data. Uni means "one", so the data has only one variable (univariate).[5] Univariate data requires to analyze each variable separately. Data is gathered for the purpose of answering a question, or more specifically, a research question. Univariate data does not answer research questions about relationships between variables, but rather it is used to describe one characteristic or attribute that varies from observation to observation.[6] Usually there are two purposes that a researcher can look for. The first one is to answer a research question with descriptive study and the second one is to get knowledge about how attribute varies with individual effect of a variable in Regression analysis. There are some ways to describe patterns found in univariate data which include graphical methods, measures of central tendency and measures of variability.[7]
Like other forms of statistics, it can be inferential or descriptive. The key fact is that only one variable is involved.
Univariate analysis can yield misleading results in cases in which multivariate analysis is more appropriate.
Measures of central tendency
Central tendency is one of the most common numerical descriptive measures. It's used to estimate the central location of the univariate data by the calculation of mean, median and mode.[8] Each of these calculations has its own advantages and limitations. The mean has the advantage that its calculation includes each value of the data set, but it is particularly susceptible to the influence of outliers. The median is a better measure when the data set contains outliers. The mode is simple to locate. The important thing is that it's not restricted to using only one of these measure of central tendency. If the data being analyzed is categorical, then the only measure of central tendency that can be used is the mode. However, if the data is numerical in nature (ordinal or interval/ratio) then the mode, median, or mean can all be used to describe the data. Using more than one of these measures provides a more accurate descriptive summary of central tendency for the univariate.[9]
Measures of variability
A measure of variability or dispersion (deviation from the mean) of a univariate data set can reveal the shape of a univariate data distribution more sufficiently. It will provide some information about the variation among data values. The measures of variability together with the measures of central tendency give a better picture of the data than the measures of central tendency alone.[10] The three most frequently used measures of variability are range, variance and standard deviation.[11] The appropriateness of each measure would depend on the type of data, the shape of the distribution of data and which measure of central tendency are being used. If the data is categorical, then there is no measure of variability to report. For data that is numerical, all three measures are possible. If the distribution of data is symmetrical, then the measures of variability are usually the variance and standard deviation. However, if the data are skewed, then the measure of variability that would be appropriate for that data set is the range.[12]
Descriptive methods
Descriptive statistics describe a sample or population. They can be part of exploratory data analysis.[13]
The appropriate statistic depends on the level of measurement. For nominal variables, a frequency table and a listing of the mode(s) is sufficient. For ordinal variables the median can be calculated as a measure of central tendency and the range (and variations of it) as a measure of dispersion. For interval level variables, the arithmetic mean (average) and standard deviation are added to the toolbox and, for ratio level variables, we add the geometric mean and harmonic mean as measures of central tendency and the coefficient of variation as a measure of dispersion.
For interval and ratio level data, further descriptors include the variable's skewness and kurtosis.
Inferential methods
Inferential methods allow us to infer from a sample to a population.[13] For a nominal variable a one-way chi-square (goodness of fit) test can help determine if our sample matches that of some population.[14] For interval and ratio level data, a one-sample t-test can let us infer whether the mean in our sample matches some proposed number (typically 0). Other available tests of location include the one-sample sign test and Wilcoxon signed rank test.
Graphical methods
The most frequently used graphical illustrations for univariate data are:
Frequency distribution tables
Frequency is how many times a number occurs. The frequency of an observation in statistics tells us the number of times the observation occurs in the data. For example, in the following list of numbers {1, 2, 3, 4, 6, 9, 9, 8, 5, 1, 1, 9, 9, 0, 6, 9}, the frequency of the number 9 is 5 (because it occurs 5 times in this data set).
Bar charts
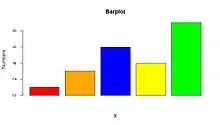
Bar chart is a graph consisting of rectangular bars. These bars actually represents number or percentage of observations of existing categories in a variable. The length or height of bars gives a visual representation of the proportional differences among categories.
Histograms
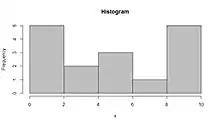
Histograms are used to estimate distribution of the data, with the frequency of values assigned to a value range called a bin.[15]
Pie charts
Pie chart is a circle divided into portions that represent the relative frequencies or percentages of a population or a sample belonging to different categories.
Univariate distributions
Univariate distribution is a dispersal type of a single random variable described either with a probability mass function (pmf) for discrete probability distribution, or probability density function (pdf) for continuous probability distribution.[16] It is not to be confused with multivariate distribution.
Common discrete distributions
Uniform distribution (discrete)
Bernoulli distribution
Binomial distribution
Geometric distribution
Negative binomial distribution
Poisson distribution
Hypergeometric distribution
Zeta distribution
Common continuous distributions
Uniform distribution (continuous)
Normal distribution
Gamma distribution
Exponential distribution
Weibull distribution
Cauchy distribution
Beta distribution
See also
References
- Kachigan, Sam Kash (1986). Statistical analysis : an interdisciplinary introduction to univariate & multivariate methods. New York: Radius Press. ISBN 0-942154-99-1.
- Lacke, Prem S. Mann ; with the help of Christopher Jay (2010). Introductory statistics (7th ed.). Hoboken, NJ: John Wiley & Sons. ISBN 978-0-470-44466-5.
{{cite book}}
: CS1 maint: multiple names: authors list (link) - Anderson, David R.; Sweeney, Dennis J.; Williams, Thomas A. Statistics For Business & Economics (Tenth ed.). Cengage Learning. p. 1018. ISBN 978-0-324-80926-8.
- Lacke, Prem S. Mann; with the help of Christopher Jay (2010). Introductory statistics (7th ed.). Hoboken, NJ: John Wiley & Sons. ISBN 978-0-470-44466-5.
{{cite book}}
: CS1 maint: multiple names: authors list (link) - "Univariate analysis". stathow.
- "Univariate Data". study.com.
- Trochim, William. "Descriptive Statistics". Web Center for Social Research Methods. Retrieved 15 February 2017.
- Stepanski, Norm O'Rourke, Larry Hatcher, Edward J. (2005). A step-by-step approach to using SAS for univariate & multivariate statistics (2nd ed.). New York: Wiley-Interscience. ISBN 1-59047-417-1.
{{cite book}}
: CS1 maint: multiple names: authors list (link) - Longnecker, R. Lyman Ott, Michael (2009). An introduction to statistical methods and data analysis (6th ed., International ed.). Pacific Grove, Calif.: Brooks/Cole. ISBN 978-0-495-10914-3.
{{cite book}}
: CS1 maint: multiple names: authors list (link) - Meloun, Milan; Militky, Jirí (2011). Statistical Data Analysis A Practical Guide. New Delhi: Woodhead Pub Ltd. ISBN 978-0-85709-109-3.
- Purves, David Freedman ; Robert Pisani ; Roger (2007). Statistics (4. ed.). New York [u.a.]: Norton. ISBN 978-0-393-92972-0.
{{cite book}}
: CS1 maint: multiple names: authors list (link) - Anderson, David R.; Sweeney, Dennis J.; Williams, Thomas A. Statistics For Business & Economics (Tenth ed.). Cengage Learning. p. 1018. ISBN 978-0-324-80926-8.
- Everitt, Brian (1998). The Cambridge Dictionary of Statistics. Cambridge, UK New York: Cambridge University Press. ISBN 0521593468.
- "One-Way Chi-Square".
- Diez, David M.; Barr, Christopher D.; Çetinkaya-Rundel, Mine (2015). OpenIntro Statistics (3rd ed.). OpenIntro, Inc. p. 30. ISBN 978-1-9434-5003-9.
- Samaniego, Francisco J. (2014). Stochastic modeling and mathematical statistics : a text for statisticians and quantitative scientists. Boca Raton: CRC Press. p. 167. ISBN 978-1-4665-6046-8.